Home
Where one teaches, two learn.
I am a web developer
I have been developing websites since 1993. In my long and storied career, my niche has been putting easy-to-use control panels between a harried user and a complicated business process. My websites have helped users find lost children, configure complex cloud storage, track endangered animals, apply for home insurance, and sequence the human genome.
I use the most modern tools available: React, Typescript, NextJS, Webworkers and WASM. I use what's useful and what my team understands, because the last thing I want is to saddle an employer with esoteric languages and processes where it's hard to find good talent.
I am a software engineer
Almost every one of my jobs has required working on the back-end. Those complex business processes come with extras-- Spiral Genetics had only the sequencer, and I wrote the server to manage both monitoring the sequencer and billing the customer. At Isilon, I wrote the servers for statistic gathering and configuration, in C++, since we had neither and the UI control panel needed both. At Indieflix, I wrote the intake server so students could upload their films without having to mail a DVD, and at MissingKids I wrote, well, everything; it was 1997 and most of the services we take for granted didn't even exist.
I use Go and Python for most of my back-end work, because those are easy to hire for. I like PostgreSQL (and Aurora) as my datastore because it's a known entity. I use my spare time to study harder things, like Haskell, Lisp, and Rust, because I believe that knowing more makes you a better developer.
I have the skills
Front-end
Web Design
Javascript
TypeScript
HTML
CSS
Web Workers
React
NextJS
Back-end
Python
NodeJS
SQL
Go
Rust
Express
Other
AWS
Test-driven Development
CI/CD
Mentorship
Documentation
Communication
I have the experience
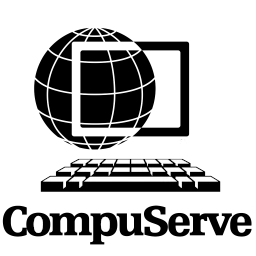
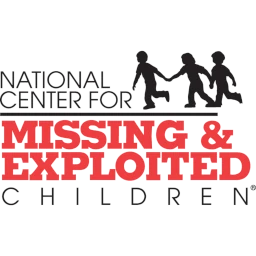
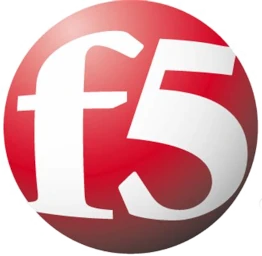
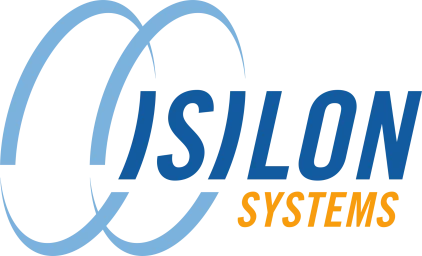
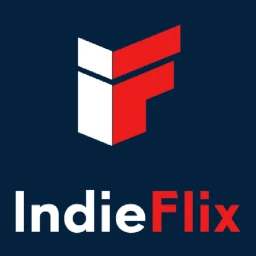
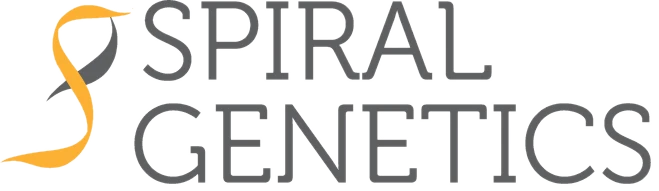
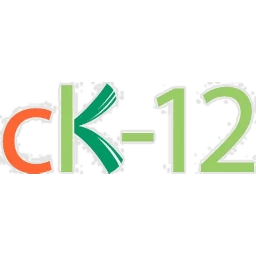
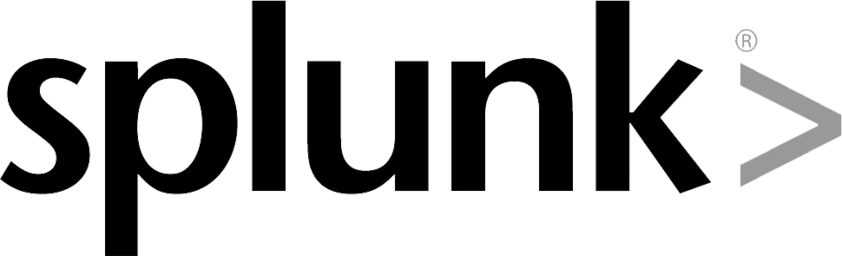
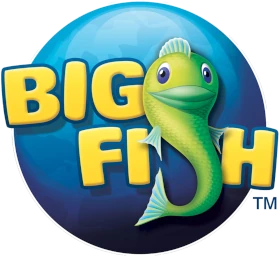
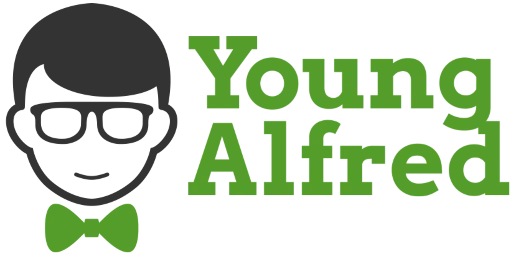